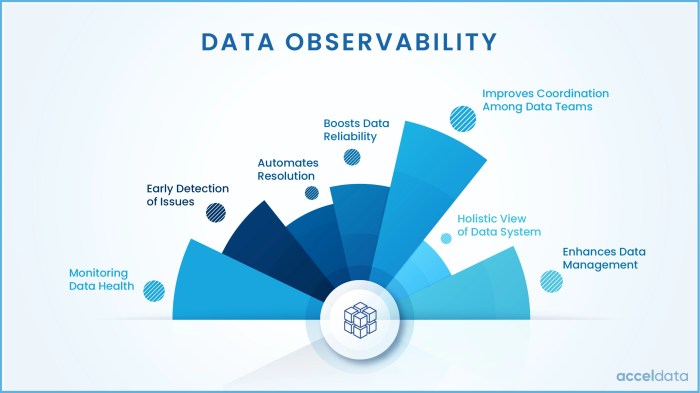
3 Trends Driving Data Observability: In today’s data-driven world, understanding and managing data is more critical than ever. As businesses increasingly rely on data for decision-making, the need for data observability has become paramount. Data observability ensures that data is accurate, reliable, and readily available, empowering organizations to make informed decisions and drive better outcomes.
This article explores three key trends that are shaping the future of data observability.
The rise of cloud-native data platforms, the increasing complexity of data pipelines, and the growing demand for data-driven decision-making are all driving forces behind the evolution of data observability. These trends are transforming how businesses manage and utilize data, creating new opportunities and challenges for data professionals.
The Rise of Cloud-Native Data Platforms: 3 Trends Driving Data Observability
The shift towards cloud-native architectures is fundamentally transforming how organizations approach data management and observability. Cloud-native data platforms offer a more agile, scalable, and cost-effective approach compared to traditional on-premises systems. These platforms are designed to leverage the inherent advantages of cloud computing, such as elasticity, pay-as-you-go pricing, and a focus on automation.
Impact on Data Observability
Cloud-native data platforms significantly enhance data observability by providing a unified and centralized view of data across the entire data pipeline. This holistic perspective empowers organizations to gain deeper insights into data quality, performance, and lineage, enabling them to proactively identify and address potential issues.
Simplifying Data Management and Observability
Cloud-native platforms simplify data management and observability by:* Streamlining Data Pipelines:Cloud-native platforms offer pre-built data pipelines and integration capabilities, reducing the need for complex custom development.
Centralized Data Governance
They provide a single platform for managing data access, security, and compliance, simplifying data governance and reducing the risk of data breaches.
Automated Monitoring and Alerts
Cloud-native platforms incorporate automated monitoring and alerting systems, enabling proactive identification and resolution of data issues.
Examples of Cloud-Native Data Platforms, 3 trends driving data observability
Several prominent cloud-native data platforms are driving the evolution of data observability:* Amazon Redshift:Amazon’s fully managed data warehouse service offers powerful analytics capabilities and built-in monitoring features, providing insights into data quality and performance.
Google BigQuery
Google’s serverless data warehouse platform provides a scalable and cost-effective solution for data analytics and observability, with features like data lineage and query performance monitoring.
Snowflake
Snowflake’s cloud-based data warehouse offers a unified platform for data storage, processing, and analysis, enabling comprehensive data observability with features like data quality monitoring and anomaly detection.
Benefits and Challenges of Cloud-Native Data Platforms
Benefits | Challenges |
---|---|
Increased agility and scalability | Vendor lock-in and potential migration challenges |
Improved cost efficiency and pay-as-you-go pricing | Security and compliance concerns in the cloud environment |
Enhanced data observability and insights | Potential dependence on cloud provider services and infrastructure |
The Increasing Complexity of Data Pipelines
Modern data pipelines are no longer simple, linear processes. They have evolved into intricate networks of interconnected components, each with its own unique characteristics and requirements. This increasing complexity stems from the growing volume, velocity, and variety of data, coupled with the rise of cloud-native technologies and the need for real-time insights.
Challenges of Managing Complex Data Pipelines
The complexity of modern data pipelines poses significant challenges for data professionals. These challenges can impact data quality, reliability, and ultimately, the effectiveness of data-driven decision-making.
- Data Source Heterogeneity:Data pipelines often ingest data from multiple sources, each with its own format, schema, and data quality. This heterogeneity makes it difficult to ensure consistency and accuracy across the entire pipeline.
- Data Transformation Complexity:As data flows through the pipeline, it undergoes various transformations, such as cleaning, enrichment, and aggregation. The complexity of these transformations can introduce errors and inconsistencies, impacting data quality and reliability.
- Pipeline Monitoring and Debugging:Monitoring and debugging complex pipelines can be a daunting task. Identifying the root cause of data quality issues or performance bottlenecks requires a deep understanding of the pipeline’s intricate flow and dependencies.
- Scalability and Performance:As data volumes grow, data pipelines need to scale effectively to handle the increased workload. Maintaining performance while ensuring data quality can be a significant challenge.
- Security and Compliance:Data pipelines often handle sensitive data, making security and compliance a top priority. Ensuring data privacy and regulatory adherence across the entire pipeline is crucial.
Best Practices for Data Observability in Complex Pipelines
To address the challenges of managing complex data pipelines, data observability solutions are becoming increasingly essential. These solutions provide real-time visibility into the health, performance, and quality of data pipelines, enabling proactive monitoring, early detection of issues, and rapid troubleshooting.
- Define Clear Data Quality Metrics:Establish specific data quality metrics that are relevant to your business needs. These metrics should be measurable and actionable, allowing you to track data quality over time and identify potential issues.
- Implement Data Lineage Tracking:Track the flow of data through the pipeline, documenting its transformations and dependencies. This lineage information is crucial for understanding data quality issues and identifying their root causes.
- Automate Monitoring and Alerting:Set up automated monitoring systems that continuously track key data quality metrics and alert you to any deviations from predefined thresholds. This allows for early detection of issues and timely intervention.
- Leverage Data Observability Tools:Utilize specialized data observability tools that provide comprehensive insights into the health, performance, and quality of your data pipelines. These tools often offer features such as data lineage tracking, anomaly detection, and data profiling.
- Embrace a Culture of Data Quality:Foster a culture of data quality throughout your organization. Encourage data professionals to take ownership of data quality and actively monitor and improve the pipelines they manage.
Data Pipeline Monitoring Tools and Capabilities
Here’s a table summarizing different data pipeline monitoring tools and their capabilities in relation to data observability:
The rise of cloud computing, the explosion of data, and the increasing complexity of data pipelines are just a few of the trends driving the need for data observability. It’s crucial to ensure data quality and reliability, and tools like those found in the new from Leigh Tucker Willow at Dunnes Stores collection can help.
These trends are pushing businesses to adopt new technologies and strategies to gain deeper insights into their data, making data observability a critical component of modern data management.
The rise of data observability is being driven by three key trends: the increasing complexity of data pipelines, the growing reliance on data-driven decision making, and the need for greater data reliability. This is evident in recent news, like clarification mirasol resources announces private placement financing , which highlights the importance of ensuring data quality and accessibility for crucial business decisions.
These trends are pushing organizations to invest in data observability solutions that provide real-time insights into data health and performance.
Data observability is exploding, driven by the need for real-time insights, the rise of cloud-native architectures, and the increasing complexity of modern data pipelines. Speaking of complexity, have you seen the amazing things people are creating with DIY projects like teether and pacifier clip diy ?
The creativity is inspiring, just like the way data observability is helping organizations gain a deeper understanding of their data and make better decisions.