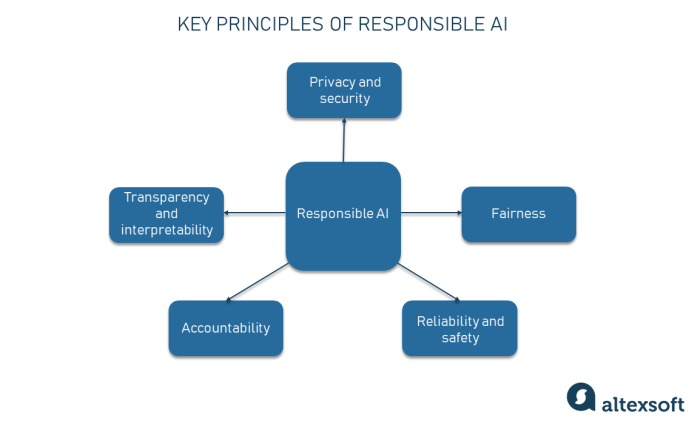
4 Principles for Responsible AI: Building Trust in the Age of Machines – as artificial intelligence (AI) becomes increasingly integrated into our lives, ensuring its responsible development and deployment is crucial. This means building systems that are not only powerful but also fair, transparent, privacy-focused, and accountable.
These four principles form the bedrock of ethical AI development, guiding us to create technology that benefits society as a whole. By understanding and adhering to these principles, we can ensure that AI remains a force for good, empowering innovation while protecting our fundamental values.
Fairness and Bias Mitigation
AI systems are powerful tools that can have a profound impact on our lives, but they are not immune to the biases that exist in the real world. In fact, AI systems can perpetuate and even amplify existing societal biases, leading to unfair and discriminatory outcomes.
Identifying and Mitigating Bias in AI Development
Identifying and mitigating bias in AI development is crucial to ensure that these systems are fair and equitable. Here are some key methods:
- Data Auditing:This involves carefully examining the data used to train AI models to identify and address any potential biases. This can include analyzing the data for imbalances in representation, checking for biased labels or annotations, and evaluating the data’s relevance to the task at hand.
- Fairness Metrics:These are quantitative measures that assess the fairness of an AI system. Common fairness metrics include accuracy parity, equalized odds, and demographic parity. By applying these metrics, developers can identify and quantify biases in the system’s predictions.
- Bias Mitigation Techniques:There are various techniques for mitigating bias in AI systems, including:
- Data Augmentation:This involves adding synthetic data to the training set to improve the representation of underrepresented groups.
- Re-weighting:This involves adjusting the weights of different data points during training to compensate for biases in the data.
- Fairness-aware Algorithms:These are algorithms designed to explicitly consider fairness during the learning process.
The Importance of Diverse Datasets and Inclusive Representation
The datasets used to train AI systems play a crucial role in determining the fairness and inclusivity of the resulting models. Diverse datasets, representing the full range of human experiences and perspectives, are essential for building AI systems that are unbiased and equitable.
Thinking about the 4 principles for responsible AI, it’s interesting to consider how they apply to something like super bomberman r online switch. While the game itself doesn’t involve complex AI, the development process could benefit from considering fairness, transparency, accountability, and privacy.
For instance, ensuring fair matchmaking and balanced gameplay would be crucial for a positive user experience.
- Real-world Examples:In the field of healthcare, AI systems trained on datasets that predominantly represent white populations may struggle to accurately diagnose conditions in patients of color. This is because the datasets may not adequately capture the nuances of different ethnicities and their health conditions.
This can lead to misdiagnosis and unequal treatment.
- Importance of Representation:AI systems should be trained on data that reflects the diversity of the population they are intended to serve. This means including individuals from different genders, races, ethnicities, socioeconomic backgrounds, and other demographic groups. By ensuring inclusive representation in training data, developers can help mitigate biases and create AI systems that are more fair and equitable for all.
Transparency and Explainability
Transparency and explainability are crucial aspects of responsible AI, ensuring that AI systems are understandable and accountable. When AI models are opaque, it becomes difficult to trust their decisions, especially in high-stakes applications.
The four principles of responsible AI – fairness, accountability, transparency, and privacy – are essential for ensuring ethical development and deployment. These principles remind me of the lessons I’ve learned from my first garden, like the importance of careful planning, understanding the needs of each plant, and the delicate balance of nature.
Just as I wouldn’t want to neglect the needs of my garden, we must ensure that AI systems are developed and used responsibly, considering the potential impact on individuals and society. What I’ve learned so far from my first garden has given me a new perspective on the importance of responsible development, and I believe these principles are equally crucial for ensuring that AI benefits everyone.
Explainability in AI refers to the ability to understand how an AI model arrives at its predictions. This is particularly important when dealing with complex models like deep neural networks, which often operate as “black boxes” where the decision-making process is not readily interpretable.
Without explainability, it becomes challenging to identify biases, errors, and ethical concerns within the AI system.
When it comes to building responsible AI, it’s crucial to consider fairness, transparency, accountability, and privacy. It’s like the old saying, “birds of a feather flock together,” meaning we should ensure AI systems don’t perpetuate existing biases or create new ones.
birds of a feather flock together – this proverb reminds us to be mindful of the potential for AI to amplify existing inequalities. By upholding these principles, we can create AI that benefits everyone, not just a select few.
Examples of AI Models Difficult to Understand, 4 principles for responsible ai
Several AI models are notoriously difficult to understand and interpret, making it challenging to assess their fairness, reliability, and ethical implications. Some prominent examples include:
- Deep Neural Networks (DNNs):These models, often used in image recognition, natural language processing, and other complex tasks, consist of multiple layers of interconnected nodes, making it challenging to pinpoint the specific factors driving a particular decision.
- Support Vector Machines (SVMs):These models, commonly employed for classification tasks, use complex mathematical functions to create decision boundaries, making it difficult to interpret the reasoning behind specific classifications.
- Random Forests:While generally considered more interpretable than DNNs, these models still involve numerous decision trees, making it challenging to trace the path leading to a specific prediction.
Methods for Making AI Decisions Transparent and Understandable
Several techniques and approaches can be employed to enhance the transparency and explainability of AI decisions. These methods aim to shed light on the inner workings of AI models, making them more understandable and accountable.
- Feature Importance Analysis:This technique identifies the most influential input features that contribute to the model’s predictions. By understanding which features carry the most weight, users can gain insights into the model’s decision-making process. This approach can be particularly useful for detecting potential biases in the data.
- Decision Rule Extraction:This method aims to extract human-readable rules from the AI model, providing a more transparent explanation of the decision-making process. By representing the model’s logic in a simplified form, users can better understand how the model arrives at its conclusions.
- Local Interpretable Model-Agnostic Explanations (LIME):LIME is a popular technique that generates local explanations for individual predictions. It works by creating a simplified, interpretable model that mimics the behavior of the original complex model in a specific region of the input space. This allows users to understand the factors driving a particular prediction without needing to understand the entire model.
- Counterfactual Explanations:These explanations provide insights into what changes would need to be made to the input data to alter the model’s prediction. By understanding the factors that influence the outcome, users can gain a deeper understanding of the model’s logic and potential biases.
Privacy and Data Security: 4 Principles For Responsible Ai
The ethical implications of data collection and utilization in AI systems are paramount. As AI models learn from vast datasets, it’s crucial to ensure that this data is collected and used responsibly, respecting user privacy and safeguarding data security.
Best Practices for Data Privacy and Security
Protecting user privacy and data security is essential in AI development. Here are some best practices:
- Data Minimization:Only collect the data necessary for the AI system’s intended purpose. Avoid collecting unnecessary personal information.
- Informed Consent:Obtain explicit and informed consent from individuals before collecting and using their data. Clearly explain the purpose of data collection and how it will be used.
- Data Encryption:Encrypt sensitive data both at rest and in transit to protect it from unauthorized access.
- Access Control:Implement robust access control mechanisms to limit access to data only to authorized individuals and systems.
- Regular Security Audits:Conduct regular security audits to identify and address vulnerabilities in data storage and processing systems.
- Data Retention Policies:Establish clear data retention policies and delete data that is no longer needed. This helps to minimize the risk of data breaches and unauthorized access.
- Privacy by Design:Incorporate privacy considerations into the design and development of AI systems from the outset. This ensures that privacy is not an afterthought.
Data Anonymization and Differential Privacy
Data anonymization and differential privacy are techniques used to protect user privacy while enabling data analysis and AI model training.
- Data Anonymization:This involves removing or modifying personally identifiable information from data sets. Techniques like generalization, suppression, and perturbation can be used to achieve anonymization. For example, replacing specific addresses with zip codes or age ranges.
- Differential Privacy:This technique adds noise to data in a way that preserves statistical properties while making it difficult to identify individual records. It ensures that the analysis results are not significantly affected by the inclusion or exclusion of any single individual’s data.
For example, adding random noise to the number of people who purchased a specific product.
Accountability and Control
The fourth and final principle of responsible AI, accountability and control, is crucial for ensuring that AI systems are developed and deployed in a way that is ethical and beneficial to society. As AI systems become increasingly sophisticated, it becomes more challenging to understand how they make decisions and to hold them accountable for their actions.
This principle focuses on establishing clear lines of responsibility and mechanisms for oversight to ensure that AI systems are used safely and responsibly.
Defining Lines of Responsibility in AI Development and Deployment
Determining who is accountable for the actions of an AI system is a complex issue. It requires careful consideration of the roles of different stakeholders involved in the AI lifecycle, from data collection and model development to deployment and monitoring.
- AI Developers:Developers are responsible for designing and building the AI system, ensuring it adheres to ethical guidelines and safety standards. They must be transparent about the system’s capabilities and limitations and provide adequate documentation and training materials.
- Data Providers:Those providing data for AI training are responsible for ensuring the data is accurate, representative, and free from bias. They must also address privacy concerns and comply with relevant data protection regulations.
- AI Deployers:Organizations deploying AI systems are responsible for monitoring their performance, identifying and mitigating risks, and responding to any adverse impacts. They must also have clear policies and procedures in place for handling ethical concerns and disputes.
- AI Users:Users of AI systems are responsible for understanding the limitations and potential risks of the system. They should be aware of their rights and responsibilities and report any issues or concerns to the relevant stakeholders.
Establishing Mechanisms for AI Governance
To effectively manage the accountability and control of AI systems, it is essential to establish robust governance mechanisms. These mechanisms should involve various stakeholders, including government agencies, industry bodies, researchers, and civil society organizations.
- Regulatory Frameworks:Governments have a crucial role in setting ethical guidelines and regulatory frameworks for AI development and deployment. These frameworks should address issues such as data privacy, algorithmic bias, and transparency. Examples include the European Union’s General Data Protection Regulation (GDPR) and the California Consumer Privacy Act (CCPA).
- Auditing and Oversight:Independent audits and oversight mechanisms are essential for ensuring that AI systems comply with ethical guidelines and regulatory requirements. These audits should assess the system’s fairness, transparency, and accountability.
- Ethical Review Boards:Ethical review boards can play a crucial role in evaluating the ethical implications of AI projects before deployment. These boards should consist of experts in AI, ethics, and relevant fields, and they should be independent of the developers and deployers of the system.
- Public Engagement and Dialogue:Open and transparent communication about AI systems is essential for building public trust and fostering responsible AI development. This includes engaging with the public to discuss the ethical implications of AI and to solicit feedback on proposed AI projects.
Roles and Responsibilities of Stakeholders in AI Governance
The following table Artikels the roles and responsibilities of different stakeholders in AI governance:
Stakeholder | Roles and Responsibilities |
---|---|
AI Developers |
|
Data Providers |
|
AI Deployers |
|
AI Users |
|
Government Agencies |
|
Industry Bodies |
|
Researchers |
|
Civil Society Organizations |
|