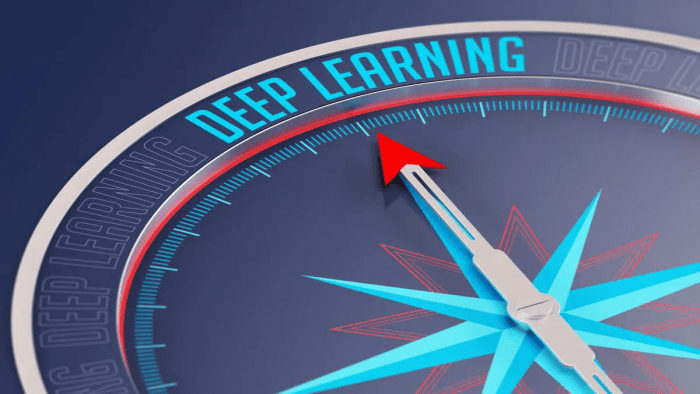
Deep instinct deep learning is a fascinating field that explores the intersection of artificial intelligence and the ancient concept of instinct. Imagine machines learning to understand and even predict our most primal urges and responses. This groundbreaking technology has the potential to revolutionize everything from healthcare to robotics, offering unprecedented insights into the workings of the human mind.
By delving into the complex interplay between deep learning algorithms and our inherent instincts, we can unlock a new understanding of how we think, feel, and behave. This field holds the key to unraveling the mysteries of human cognition and unlocking the potential for a future where machines can not only understand but also anticipate our needs and desires.
Deep Instinct: Deep Instinct Deep Learning
Deep Instinct is a revolutionary approach to cybersecurity that leverages the power of deep learning to anticipate and prevent cyberattacks. This technology goes beyond traditional security measures by analyzing vast amounts of data to learn the patterns and characteristics of malicious activities.
By understanding these patterns, Deep Instinct can proactively identify and block threats before they even reach their targets.
Deep Instinct: Key Principles and Methodologies
Deep Instinct is based on the principles of deep learning, a branch of artificial intelligence that uses artificial neural networks to analyze complex data. The core methodologies behind Deep Instinct include:
- Deep Neural Networks:Deep Instinct utilizes deep neural networks, which are complex structures inspired by the human brain. These networks consist of multiple layers of interconnected nodes that process information in a hierarchical manner. By analyzing data from various sources, these networks learn to identify patterns and anomalies that indicate malicious activity.
Deep instinct deep learning is all about mimicking the way our brains work, but sometimes I need a break from the complex algorithms and dive into something delicious. A recent culinary adventure led me to a basil cheesecake with chocolate sauce recipe, which, surprisingly, was a perfect match for my need to escape the world of artificial intelligence.
It was a sweet and savory experience that reminded me of the importance of balancing intellectual pursuits with simple pleasures.
- Unsupervised Learning:Unlike traditional machine learning approaches that rely on labeled data, Deep Instinct employs unsupervised learning techniques. This means the system learns from unlabeled data, discovering patterns and relationships without explicit instructions. This approach allows Deep Instinct to adapt to evolving threats and identify previously unknown attack methods.
Deep instinct deep learning is all about understanding the subtle nuances of human behavior, those gut feelings that guide our decisions. It’s like a detective uncovering hidden clues, piecing together patterns to predict what might happen next. This type of learning is particularly relevant to organizations like the Tate, who are constantly striving to understand their members’ preferences and engagement, as evidenced by their annual member survey.
By analyzing data on visitor behavior, the Tate can better tailor their programming and exhibitions to resonate with their audience, ensuring a more enriching and engaging experience for everyone.
- Continuous Learning:Deep Instinct is constantly learning and evolving, continuously analyzing new data to refine its detection capabilities. This continuous learning process ensures that the system remains effective against new and emerging threats.
Applications and Benefits of Deep Instinct
Deep Instinct offers a wide range of applications and benefits across various fields, including:
- Endpoint Security:Deep Instinct provides robust endpoint protection by identifying and blocking malware before it can execute on devices. This proactive approach helps prevent data breaches and ensures the security of sensitive information.
- Network Security:By analyzing network traffic, Deep Instinct can detect and prevent advanced threats, including zero-day attacks, that traditional security solutions might miss. This helps organizations maintain a secure network environment and protect against cyberattacks.
- Cloud Security:Deep Instinct safeguards cloud environments by identifying and neutralizing threats targeting cloud infrastructure and applications. This ensures the security and integrity of data stored and processed in the cloud.
- Threat Intelligence:Deep Instinct provides valuable threat intelligence by analyzing vast amounts of data to identify emerging threats and trends. This information helps organizations stay ahead of attackers and proactively mitigate risks.
Real-World Examples of Deep Instinct
Deep Instinct has been successfully deployed in various real-world scenarios, demonstrating its effectiveness in protecting against cyberattacks. For example, Deep Instinct has helped organizations prevent ransomware attacks, block phishing campaigns, and neutralize advanced malware threats.
“Deep Instinct is a game-changer for cybersecurity. It’s the first solution that can truly anticipate and prevent attacks before they happen.”
Deep instinct deep learning is a fascinating field, exploring how our subconscious biases and learned patterns influence our decisions. It’s like a hidden layer of neural networks, constantly processing information and shaping our perceptions. And just like our minds evolve and adapt, so do trends in fashion.
This spring and summer, spring summer maple jewelery is here , a beautiful example of how natural elements inspire contemporary design. This connection between nature and human expression is something that deep instinct deep learning aims to understand and unlock.
[CEO of a major cybersecurity company]
Deep Learning for Instinctual Behavior
Deep learning, a powerful subset of artificial intelligence, has emerged as a promising tool for modeling and understanding instinctual behavior. This approach leverages the ability of deep neural networks to learn complex patterns from vast datasets, providing insights into the underlying mechanisms driving instinctive actions in various species.
Deep Learning Models for Analyzing and Predicting Animal Instincts
Deep learning models have been successfully applied to analyze and predict animal instincts, providing valuable insights into their behavior and decision-making processes. These models can be trained on large datasets of animal behavior, including video footage, sensor data, and environmental information.
- Predator-Prey Interactions:Deep learning models have been used to analyze the interactions between predators and prey, identifying patterns in their movements and behaviors. These models can predict the likelihood of a predator attacking a prey animal based on factors such as distance, speed, and environmental conditions.
- Navigation and Spatial Memory:Deep learning models have been used to study the navigational abilities of animals, such as birds and insects. By analyzing their flight patterns and sensory inputs, these models can identify the underlying mechanisms that enable them to navigate complex environments.
- Social Behavior:Deep learning models have been applied to analyze the social interactions of animals, such as flocking behavior in birds or group dynamics in primates. These models can identify patterns in social interactions and predict how individuals will respond to different stimuli.
Comparing Approaches for Deep Instinct Modeling in Different Species
The approaches used for deep instinct modeling vary depending on the species and the specific instinct being studied. Some common approaches include:
- Reinforcement Learning:This approach involves training deep learning models to learn optimal behaviors through trial and error. This is particularly useful for studying instincts that involve learning and adaptation, such as foraging behavior in animals.
- Generative Adversarial Networks (GANs):GANs are a type of deep learning model that can generate realistic data, such as images or videos. This approach has been used to study the visual perception of animals, such as the ability of birds to recognize predators or the ability of insects to navigate using visual cues.
- Convolutional Neural Networks (CNNs):CNNs are a type of deep learning model that is particularly well-suited for processing images and videos. These models have been used to analyze the visual stimuli that trigger instinctive behaviors, such as the flight response in birds or the startle response in mammals.
Challenges and Future Directions
Despite the significant progress in deep learning for instinctual behavior, there are still challenges to be addressed.
- Data Availability:The availability of large, labeled datasets of animal behavior is essential for training effective deep learning models. However, collecting such datasets can be time-consuming and expensive.
- Interpretability:Deep learning models can be complex and difficult to interpret. Understanding the specific mechanisms by which these models make predictions is crucial for gaining insights into the underlying biological processes.
- Generalizability:Deep learning models are often trained on specific datasets and may not generalize well to new situations or species. Further research is needed to develop models that are more robust and generalizable.
Deep Instinct in Human Cognition
The concept of deep instinct, as applied to artificial intelligence, has intriguing implications for understanding human cognition and decision-making. Deep instinct, in this context, refers to the ability of AI systems to learn and make decisions based on patterns and insights gleaned from massive datasets, often without explicit programming or rule-based systems.
This approach mimics the way humans often make decisions, relying on intuition and subconscious patterns learned from experience.
The Potential of Deep Instinct for Understanding Human Cognition
Deep instinct offers a new lens through which to examine the complexities of human cognition. By studying how deep learning algorithms learn and make decisions, researchers can gain insights into the underlying processes that drive human thought and behavior. This approach could lead to a deeper understanding of:
- Implicit biases:Deep learning models are known to pick up on biases present in their training data. This phenomenon mirrors the way humans can develop implicit biases, often without conscious awareness. Studying these biases in AI models could shed light on how such biases form and persist in human cognition.
- Decision-making under uncertainty:Deep instinct algorithms often excel at making decisions in complex and uncertain environments, relying on probabilistic reasoning and pattern recognition. This parallels the way humans navigate uncertainty in their daily lives, relying on intuition and past experiences. By analyzing the decision-making processes of deep learning models, researchers can gain insights into the strategies humans employ in similar situations.
- The interplay between conscious and unconscious processes:Deep instinct models highlight the role of unconscious processing in decision-making. This aligns with the understanding that human cognition is not solely driven by conscious reasoning but also influenced by subconscious processes. Studying the interplay between conscious and unconscious elements in AI models could offer valuable insights into the workings of the human mind.
A Thought Experiment: Deep Instinct and Conscious Reasoning, Deep instinct deep learning
Imagine a scenario where a deep learning model is tasked with predicting the outcome of a chess game. The model, trained on a vast dataset of chess games, develops a deep understanding of the game’s dynamics and strategic patterns. Now, consider a human chess player facing the same situation.
The human player might employ conscious reasoning, analyzing the board position and potential moves, while also relying on intuition and experience. To investigate the interplay between deep instinct and conscious reasoning, we can ask the following questions:
- How does the AI model’s decision-making process differ from the human player’s?Does the AI rely primarily on pattern recognition and probabilistic reasoning, while the human player utilizes both conscious reasoning and intuitive insights?
- Can the AI model explain its reasoning behind a particular move, while the human player might struggle to articulate the basis for their intuition?This could highlight the different ways in which conscious and unconscious processes contribute to decision-making.
- What are the strengths and weaknesses of each approach?Does the AI model excel at recognizing complex patterns, while the human player possesses a greater ability to adapt to unexpected situations?
Implications for Human Psychology and Behavior
The insights gained from studying deep instinct could have significant implications for understanding human psychology and behavior. By examining the mechanisms underlying deep instinct in AI models, researchers can gain a deeper understanding of:
- The nature of intuition:Deep instinct models offer a potential framework for understanding the origins and workings of human intuition. By studying how AI models develop intuitive insights, researchers can gain insights into the cognitive processes that underlie human intuition.
- The role of experience in shaping behavior:Deep instinct models are trained on vast datasets of past experiences, which allows them to learn and make decisions based on patterns and insights gleaned from these experiences. This parallels the way humans learn from their experiences, shaping their behavior and decision-making processes.
- The potential for bias in human cognition:Deep instinct models can be susceptible to biases present in their training data. This raises important questions about the potential for bias in human cognition, as our experiences and the information we are exposed to can shape our perceptions and decision-making.